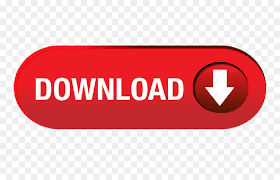

This will require repeated measurements of the x variable in the same individuals, either in a sub-study of the main data set, or in a separate data set. In general, methods for the structural model require some estimate of the variability of the x variable. (1995) give more detail on regression dilution in nonlinear models, presenting the regression dilution ratio methods as the simplest case of regression calibration methods, in which additional covariates may also be incorporated. Rosner (1992) shows that the ratio methods apply approximately to logistic regression models. Hughes (1993) shows that the regression dilution ratio methods apply approximately in survival models. Fuller (1987) is one of the standard references for assessing and correcting for regression dilution. The reply to Frost & Thompson by Longford (2001) refers the reader to other methods, expanding the regression model to acknowledge the variability in the x variable, so that no bias arises. The term regression dilution ratio, although not defined in quite the same way by all authors, is used for this general approach, in which the usual linear regression is fitted, and then a correction applied. Frost and Thompson (2000) review several methods for estimating this ratio and hence correcting the estimated slope. Under certain assumptions (typically, normal distribution assumptions) there is a known ratio between the true slope, and the expected estimated slope. For example, in a medical study patients are recruited as a sample from a population, and their characteristics such as blood pressure may be viewed as arising from a random sample. The case that the x variable arises randomly is known as the structural model or structural relationship. The case of a randomly distributed x variable It can be corrected using total least squares and errors-in-variables models in general. The case that x is fixed, but measured with noise, is known as the functional model or functional relationship. Regression slope and other regression coefficients can be disattenuated as follows.

1.2 The case of a randomly distributed x variable.The greater the variance in the x measurement, the closer the estimated slope must approach zero instead of the true value. However, variability, measurement error or random noise in the x variable causes bias in the estimated slope (as well as imprecision). Statistical variability, measurement error or random noise in the y variable causes uncertainty in the estimated slope, but not bias: on average, the procedure calculates the right slope. Regression dilution, also known as regression attenuation, is the biasing of the linear regression slope towards zero (the underestimation of its absolute value), caused by errors in the independent variable.Ĭonsider fitting a straight line for the relationship of an outcome variable y to a predictor variable x, and estimating the slope of the line. Note that the steeper green and red regression estimates are more consistent with smaller errors in the y-axis variable. Green reference lines are averages within arbitrary bins along each axis. By convention, with the independent variable on the x-axis, the shallower slope is obtained. The steeper slope is obtained when the independent variable is on the ordinate (y-axis). The shallow slope is obtained when the independent variable (or predictor) is on the abscissa (x-axis). Two regression lines (red) bound the range of linear regression possibilities. Illustration of regression dilution (or attenuation bias) by a range of regression estimates in errors-in-variables models.
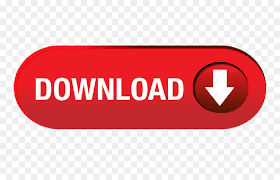